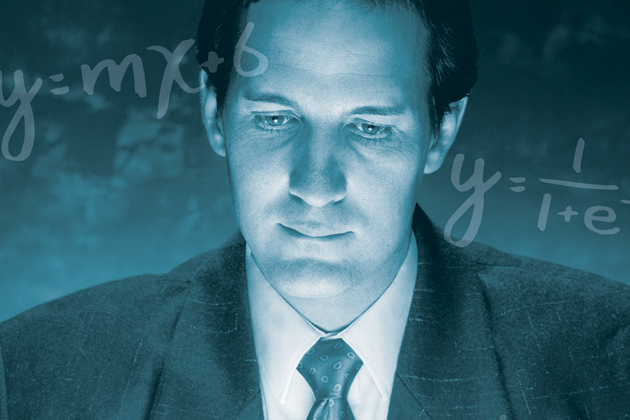
For many risk managers and claims professionals, the use of predictive analytics is a complex subject, especially since there is a lack of standard methodologies. The lines between true predictive analytics and data analysis and data access are often blurred. Terms are interchanged and performance is unsubstantiated. As a result, there is often confusion, which can hinder a risk professional’s evaluation of an algorithm’s efficacy. Becoming versed in key aspects of data and statistics, as well as knowing what to look for when evaluating a predictive analytics algorithm, can arm the risk manager with the information needed to properly evaluate, apply and use predictive analytics.
Start with the Business Problem
The goal of predictive analytics is to generate information that will help make better decisions. Therefore, it is important to build the predictive analytics algorithm with a specific business problem in mind. What is the nature of the business problem you are trying to solve? What is the outcome you hope to achieve? For example, if you are seeking an algorithm that, when put into practice, will help avoid claim losses greater than $1 million, it is not necessary to build a model to discern the dollar value of losses less than that amount.
Keeping the business problem in mind right from the start can also help determine if more than one algorithm is necessary. For example, it is often important to measure the full lifetime value of a customer, not just the initial conversion to the business. Customers who are most easily converted may not be the most easily retained. In this case, it might be best to have two predictive models: one to predict conversion and another to predict retention.
Consider Your Data Set
Data is the foundation for predictive analytics. To qualify as “predictive analytics,” an algorithm must rely upon a sufficient quantity of data to determine the best predictors for the most accurate predictions. Consider Google: By analyzing the data entered in their search engine, they accurately predict trends. In fact, Google can estimate flu levels around the world by adding all flu-related search queries together. Year after year, the company compares its model’s estimate to traditional flu surveillance systems and refines the model to improve performance. One report even suggested that Google can detect regional flu outbreaks 10 days faster than the Centers for Disease Control.
In workers compensation, pharmacy data can be used to determine which injured workers are most likely to experience high pharmacy costs or have the longest duration of opioid use. By using historical pharmacy data, you can correlate each independent factor known about the injured workers to long-term severity. With statistical modeling, these balance against each other to generate an accurate prediction. Therefore, when evaluating a predictive analytics algorithm, always consider the data set. The more data the algorithm uses, the more accurate the predictions generated.
Geography Matters
It is just as important to consider the geography as it is to consider the data set. In workers compensation, for example, every state has different regulations. One state’s solution or regulation may not work in another state for many reasons, including some specific to their geography. This is a very old problem with the use of applied statistics that is still the case today. Franz Kafka, who was a workers compensation attorney before finding fame as an author, faced the problem of applying data from Germany as he tried to manage the Austrian system. In his case, he called the data from another geography “defective and inadequate.”
Using data appropriate to your geography avoids what Kafka scholars call “a practice of calculating with dubious figures”—the kind of illogical endeavor that might now be called “Kafkaesque.”
Keep Time and Interactions in Mind
One of the greatest limitations in predictive analytics is the assumption that the future will always resemble the past. If you have built a model with data from 2010 to 2014 and you are trying to predict how long it will be before someone injured in April 2015 will return to work, there may be something unique about people injured in April 2015 that is different from those in your collected data. If the model had originally been built on data from 2010 to 2013 and tested on information from 2014, this would at least show which effects from 2010 to 2013 are still valid through 2014 (although this could overstate the significance of 2014). This helps to understand how predictors change over time. The data miners Michael Berry and Gordon Linoff call this type of approach an “out of time” test set.
Another common pitfall is using only current data. A business leader may think that the business has changed too much, making data invalid after a certain period. Most analysts, however, prefer to use as much data as possible. Depending on how important the business cycle is to your predictions, you may want a model that includes at least two recessions in your data set. In general, the time period of data collection matters if the predictors in the model change during that period. For example, the most expensive workers compensation injuries do not change over time. Spinal injuries are more severe than foot injuries—this was true in 1980, and it is still true in 2015. But other predictors may not be the same, such as the regulatory environment. If this is the case, you may be overstating the length of time an injured worker will be out of work if the regulatory environment previously favored the injured worker, but now favors the employer.
It is also very important to be aware of interactions. An analyst needs to know what in the environment has changed over time, such as whether there is something inherent in the type of injuries in a given state that is different from the rest of the country. This could overstate or understate injury severity if the mix of injuries is different or if they are treated differently.
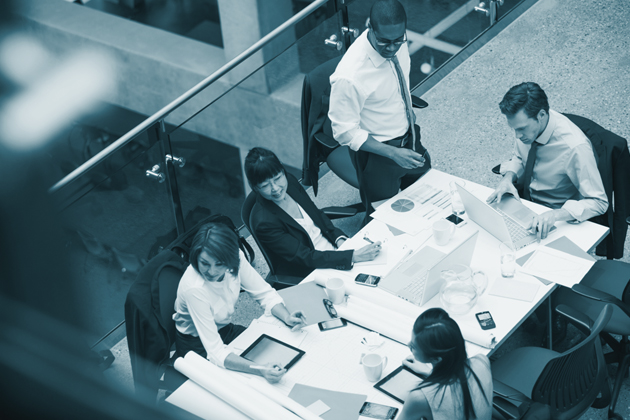
Evaluate Fully
If you have ever taken a statistics course, you may remember the term “r-squared,” which is an overall statistical performance measure for a regression model. The higher the r-squared value, the better the model. You may also remember significance tests, which allow you to be 95% confident that something is accurate. R-squared and statistical significance are important, but they are not the final test for determining accuracy.
Traditional statistical measures like r-squared assume that the decision-makers are acting at random. In workers compensation, this would mean that the risk managers and/or claims professionals have no idea which injured workers need help. This is almost never the case. Instead, compare your current decision-making process to what would be done without a predictive analytics algorithm. In a pharmacy, for example, preventing opioid misuse and abuse can be attempted simply by identifying those with injuries who already have the highest current opioid use, measured in morphine equivalents. A predictive analytics model would therefore need to demonstrate that it is better at identifying long-term use of opioids than these simple metrics. This can usually be quantified: the “simple version” is an improvement over choosing at random. Then, how much better is the predictive model than the simple version? For example, if you are showing me an r-squared for a predictive model, what is the r-squared for what I am already doing today?
In the best-case scenario, businesses are able to invest in experiments to test the predictive models in a controlled environment before implementing them across the business. If you are fortunate enough to be able to do this, you should take the time to design the experiment correctly. The most important point from an analytical perspective is to have a sufficient control group. Control groups ideally have the best decision-making tools available outside of the predictive analytics algorithm. Often, this is not just good methodology—it is ethically mandatory. As R.A. Bailey wrote in Design of Comparative Experiments, “For a given illness, if there is already a standard drug that is known to be effective, then it is not ethical to give no treatment in a clinical trial of a new drug for that illness. The control treatment must be the current standard drug.” This is similar to the point about why r-squared is insufficient: R-squared typically measures the model’s performance against random decision-making. But hardly any business processes are random. The new algorithm must be compared to the old algorithm.
At the end of the experiment, the control group can be compared to the group where the predictive analytics algorithm was applied. This is usually done through a test of statistical significance. The standard methodology is to measure the outcomes for the control group against those in the program you are trying to measure. Ideally, the outcome metric (for example, reduction in costs or duration of claim) can be quantified for both the control group and the group in the program, and they can be compared through a significance test. One of the most critical evaluators, the U.S. Food and Drug Administration, generally requires a study at the 99.875% confidence level to pass a clinical trial for a new medication, while the average business is comfortable with 80% to 95% confidence.
Focus on Comparisons
When you hear someone say, “Our model has a statistical significance of 95%,” what does that really mean? Always remember that a number by itself is never statistically significant—it must be compared to something else.
Discussions about statistics can be challenging for risk managers, but discussions about comparisons usually are not. Therefore, ask what the comparison is for the 95% significance. An appropriate answer might be something like, “Our outcomes are statistically significant because our model resulted in claims with lower costs than the control group with 95% confidence.” This brings the discussion away from numbers into something that non-statisticians are generally more comfortable talking about, such as how the control group was structured and what metrics were analyzed in each group. If these answers are satisfactory, then the risk manager can feel more comfortable with the significance test.
Continually Test Performance
While analysts will not usually be able to run a real-world experiment on their predictive models, there are still best practices they can follow. Analysts use very large data sets to build predictive models. To test the algorithm, an analyst can “partition” this data set into two or more data sets: one (or more) to build the algorithm, and a final data set to test it. The concept here is that all the statistical measures used to generate the algorithm are applied before using the final data set. Then, after building the model, it is tested on the remaining data. This simulates a real-world application of the model and helps avoid the problems of “over-fitting.”
It is critical that this testing does not just happen once. Rather, algorithms should be continually tested and updated when necessary. For example, if a model predicts an average claim cost of $100,000 but the actual average is $75,000, it is probably worth looking at why the model is constantly generating higher average predictions. You may find that something in your business has changed, and that the model is directing you to focus resources on a problem in the business that has already been solved.
Identify Trends Earlier
Predictive analytics and other algorithms are tools to help risk and claims professionals make better decisions. Their use provides the ability to identify trends earlier and prepare to take action sooner. Knowing what to look for when evaluating their performance helps determine if your business is reaping all the benefits of the latest advances in technology, and if you are appropriately addressing the business problems at hand.