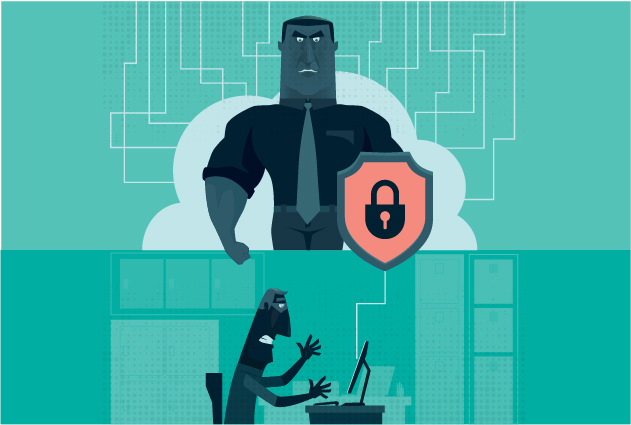
Javelin Strategy & Research reported that there were 13.1 million victims of identity fraud in 2015 and that new account fraud will increase 44% between 2014 and 2018, rising to a projected $8 billion. While these numbers are alarming, technological advancements have actually made fraudsters’ jobs more difficult when it comes to in-person transactional fraud. The bad guys do not give up very easily, though. The anonymity provided by online channels has made opening new accounts for credit cards and other non-secured loans one of the most popular methods for fraudsters.
Combine this with the easy access to personally identifiable information provided by increasingly frequent data breaches and organizations have a serious threat on their hands. Using stolen and made-up information, fraudsters can open new accounts and then focus on card-not-present transactions to steal goods and rack up unpaid loans.
“True name fraud”—when criminals use a real person’s information to open new accounts—can go undetected for months if the victim is not constantly on the lookout for suspicious activity. As a result, organizations must make every possible effort to reduce fraud and the associated loss from lawsuits, reputation damage and victim costs.
Fraud tools that simply verify the legitimacy of the name, date of birth, Social Security number and address of the person applying for an account will not stop fraud when a criminal already has information through other means of identity theft, however. Combatting true-name fraud requires a combination of tactics aimed at deciding whether the applicant is the person he or she claims to be.
To catch criminals engaging in true-name identity fraud and decrease fraud losses, a combination of authentication and modeling techniques is needed.
Predictive modeling tools can decide in real-time whether an account applicant requires additional verification. Ideally, a model will draw upon as many different data points as possible, including verifying and linking personally identifiable information to ensure that all of the information is correct and that it matches a real identity.
The ideal model should then go beyond identity factors to analyze attributes such as the channel and time of application as well as velocity of the transaction and note any suspicious behavior outside of the norm for the claimed applicant. If all information is correct and consistent, the model will say an applicant is low risk and they will keep going through the process without invasive security steps.
This keeps legitimate customers happy because they are not being hassled, and benefits the organization because it has not wasted money authenticating a low-risk transaction.
If the model flags the application for additional review, another authentication process will be initiated. Depending on the situation, tools like two-factor authentication, which delivers one-time passcodes to a user’s device, and dynamic knowledge-based authentication, which asks for information not publicly available, can help determine the legitimacy of an application.
Newer techniques like device recognition, which analyzes previous transactions on the device used to determine the likelihood of future fraud attempts, and user biometrics—voice recognition, fingerprints or facial recognition—that match the claimed identity against a known physical characteristic, round out the authentication process.
Companies should also consider certain key steps to increase their chances of successfully fighting fraud:
Define fraud. Organizations need to strictly define what constitutes fraud and make sure it is correctly classified. If you are not measuring the right thing, you cannot properly address it. This should evolve into a continual learning and improving process.
Use consortiums. Fraud consortiums are beginning to take center stage when it comes to using data to fight fraud. There has always been cooperation among fraud professionals, but as technology advances and it becomes more apparent just how valuable data is, professionals across all industries are becoming more likely to share data about fraudulent activity aimed at their organizations.
Incorporate algorithms. When organizations take advantage of proprietary algorithms to supplement their anti-fraud efforts, they can more effectively catch fraud and decrease false positives.
Hire the right people. When building your fraud team, hire curious people who relish going through data and finding patterns. Criminals are creative, so your team needs to be as well.