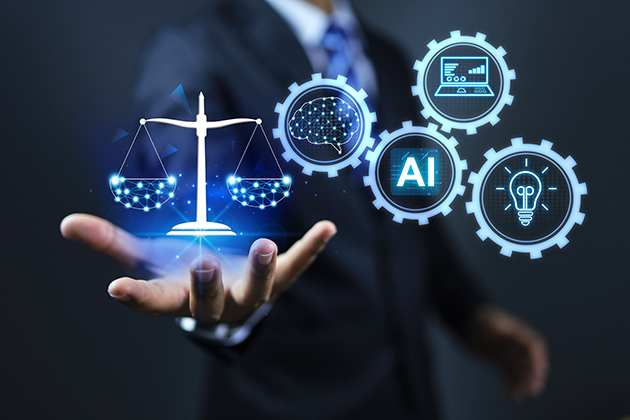
In 2024, enterprise AI use skyrocketed by 595%, reshaping industries and sparking global regulatory debates. According to the Organization for Economic Co-operation and Development (OECD), there are currently more than 1,000 AI regulations and initiatives under consideration across 69 countries. The rapid rate of AI adoption challenges lawmakers, governing boards and risk managers to balance policies that support AI development while ensuring systems are trustworthy, fair, transparent and secure.
Security and data privacy are at the root of many potential benefits and concerns around AI. According to a Lakera security trends overview, while 93% of security professionals say that AI can ensure cybersecurity, 77% of organizations find themselves unprepared to defend against AI threats. Compounding the challenge are enterprise decision-makers’ varying degrees of trust toward AI technologies. In ABBYY’s recent State of Intelligent Automation Report–AI Trust Barometer, 50% of those who do not trust AI cite concerns about cybersecurity and data breaches. Further, 47% and 38% have worries about the accuracy of the interpretation and analysis of their AI models.
When implementing AI, trust and reliability are paramount for companies, governments and institutions worldwide. For enterprise leaders, the challenge lies in navigating AI's transformative potential while managing its inherent risks.
The following four considerations can help companies ensure honest, trustworthy and credible AI policies and practices that can meet regulatory standards and manage enterprise risk.
1. Start with Good Quality Data
As the saying goes, garbage in, garbage out. AI relies on training data, much of it untouched by humans. While this is a key reason many businesses adopt it, this data is also a potential security weakness. Outdated and unstructured information or personal data is often inadvertently fed into large language models (LLMs), leading to bias, inaccuracies and opportunities for data theft and discrimination.
LLMs work best when the data they learn from is rich and well-organized. For most organizations today, this means LLMs should have a minimal understanding of the specific needs and operations they are expected to support. Retrieval augmented generation (RAG), a technique to enhance the accuracy and reliability of LLMs, is re-emerging to bridge this gap. RAG is quickly gaining traction as a cost-effective and secure method to enrich LLMs with organizational data, thus significantly enhancing their utility and trustworthiness.
To address the challenge of poor training data, organizations should begin by auditing their data repositories to identify gaps, inaccuracies and potentially sensitive information. Next, it is critical to establish clear data cleansing and structuring protocols to ensure higher-quality inputs. Relevant and well-organized data paves the way to fully leverage a RAG solution and provide LLMs with greater contextual understanding and increased business alignment. Finally, cross-functional teams can collaborate to implement RAG processes securely, ensuring robust data governance practices that safeguard against breaches.
2. Ensure Explainable AI and Transparent AI
AI-driven decisions can present biases within data, which can potentially perpetuate discrimination and inequality. To address this, it is important to have diverse teams with a broad range of perspectives shaping their capabilities developing and deploying AI systems to ensure explainable and transparent AI. Companies should implement cross-functional teams dedicated to AI ethics, including algorithmic risk management and data governance committees.
There must be emphasis on tools that enable AI transparency, bias reduction and audit trails, allowing companies to trust their AI solutions and verify compliance on demand. AI-powered security tools benefit from transparency, allowing analysts to understand decisions and improve breach detection methods.
Developers should be able to provide interfaces that allow stakeholders to understand, interpret and challenge AI decisions, especially in critical sectors like insurance, healthcare and finance. Organizations must be clear about the AI’s operation, especially when personal data is involved. When users understand how AI handles data, they can make informed choices about sharing information, reducing the risk of exposing unnecessary personal details.
Companies can prioritize accountability by creating frameworks for identifying and mitigating bias, regularly auditing their systems, and being receptive to feedback. External audits are becoming a popular way to provide an impartial perspective. For example, ForHumanity is a not-for-profit organization that can independently audit AI systems to analyze risk. They recently launched an AI Policy Accelerator that encourages AI companies to submit their products for auditing against rigorous risk management standards, providing an avenue to proactively test AI tools for regulatory compliance and responsibility.
Another path for trustworthy AI is introducing specialized or purpose-built AI such as small language models (SLMs). Enterprises have begun to pivot to purpose-built AI for specific tasks and goals. This approach cuts back on unnecessary generality to yield more efficient, accurate results while reducing risks of inaccuracy or excessive research. By compressing the model itself, the precisions of its calculations are smaller, increasing speed and accuracy.
3. Incorporate Human Oversight
AI should enhance human abilities, not replace them, especially in security management, where AI's decisions can have significant consequences. Human oversight helps keep AI systems aligned with ethical standards and societal values. Without human intervention, AI systems can make mistakes, show prejudice and be misused, leading to serious security and privacy issues.
Often referred to as human-in-the-loop (HITL), this collaborative approach combines human input with machine learning to improve the accuracy and credibility of AI systems. Organizations can incorporate HITL into different processes, including: training, where humans label training data to adjust algorithms; testing, where humans provide feedback on the model’s performance; or decision-making, where humans review and confirm AI-flagged content.
To effectively implement HITL approaches in AI systems, organizations should integrate human oversight in training, testing, deployment and maintenance phases. Teams need to label training data, provide ongoing feedback, and validate AI-generated outputs to enhance system accuracy and credibility.
It is also crucial to educate employees about AI ethics, regulatory requirements and best practices. Business leaders should provide ongoing training sessions to inform staff about changes in AI regulations and compliance strategies. Using humans to oversee ethical practices will prioritize privacy by design, ensuring that data collection, processing and storage follow secure and transparent practices.
4. Conduct Continuous Evaluation
Emerging threats and shifting regulatory landscapes pose a critical challenge for businesses, but AI can help alleviate the burden by automating regulatory monitoring. AI tools such as process intelligence (PI) can analyze workflows and large datasets to flag potential compliance issues, ensuring adherence to regulations while reducing manual efforts and costs.
Using predefined trust metrics, the software can help to detect and address risk early and prevent cyber threats before they lead to data breaches. It provides alerts when rules are broken or processes are deviated from, enabling companies to proactively address discrepancies and ensure compliance. Examples are continuous audits of financial workflows against the rules of GDPR or refining fraud detection models to reduce false positives and detect new fraud patterns. Organizations can also use it to ensure that only authorized employees can access sensitive data by tracking login.
Effective AI governance and best practices not only mitigate enterprise risks but also drive tangible business benefits, such as enhanced brand loyalty and increased customer retention. By implementing trustworthy AI frameworks, organizations can foster confidence among stakeholders, ensuring sustainable growth while safeguarding their reputation in an increasingly AI-driven world.