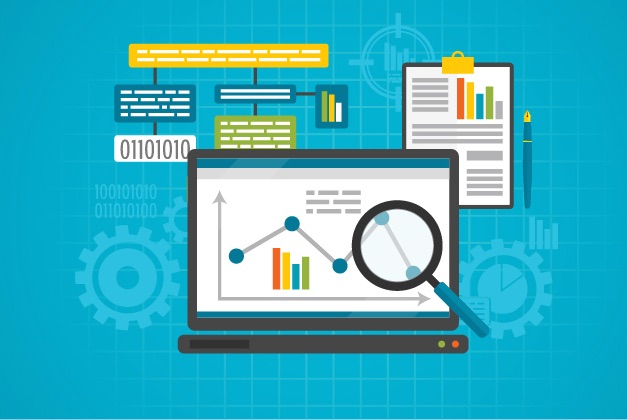
Headless worms, jailbreaks, madware, man-in-the-middle, ransomware, ghostware, watering holes and malvertising. These colorful names belie the seriousness of the security attacks plaguing organizations that continue to grow in number and sophistication.
In 2015, the Identity Theft Resource Center reported that the percentage of breaches caused by hacking was at a nine-year high. Hackers frequently lurk on networks for weeks or months—sometimes years—before detection. Indeed, the 2016 Trustwave Global Security Report found the mean time between intrusion and detection in 2015 was 80.5 days. These delays give hackers plenty of time to fulfill their mission, be it stealing intellectual property, accessing personally identifiable information, or interrupting business operations.
As organizations work to mitigate these business risks, IT security has become a significant focus of governance, risk and compliance (GRC) efforts. The supporting technology and operational workflow for setting controls and adhering to a risk-based framework is accelerating the evolution from disjointed point solutions to enterprise security platforms.
When organizations undertake the GRC process for security, they must first evaluate risks inherent in their overall infrastructure, networks and security functions. They can then develop mitigation strategies that reflect the risk and business value of the data, assets and services they are looking to protect.
Historically, assessing risks within network segments, data and network assets has been difficult because organizations have lacked a deep understanding of how their networks behave. Although network security solutions generate vast quantities of data, data quality issues, coupled with organizational and data silos, have made these solutions difficult to use. Without innovative security analysts who have institutional knowledge and data science capabilities, identifying suspicious and risky network behavior has been difficult.
Security information and event management (SIEM) and log management tools are increasingly used to centralize security and risk data to provide greater visibility. Yet despite greater data centralization, the strategy to attain greater visibility has failed to fully identify complex security hazards. The data remains uncorrelated and unable to fully identify complex security hazards unless security analysts know the specific series of questions to ask. As a result, organizations remain reactive, using solutions and resources that model known behavior patterns to identify evidence of attacks and potential breaches. Unfortunately, new threats that do not conform to existing threat signatures continuously emerge and successfully evade these tools.
Combating evolving threats requires organizations to gain a holistic view of their network, including all of the security measures in place, and to establish a baseline of activity on the network. Unless normal activity is understood, organizations cannot identify the abnormal. Increasingly, organizations are coming to realize that behavioral analytics can enable them to understand normal operations and that abnormal activity might indicate an adversary’s presence.
Advanced analytic capabilities are a must in any enterprise security platform. Yet all too often analytics is simply a buzzword, overlooking important analytic implementation and lifecycle management considerations.
So how do you ensure that analytics becomes a true force multiplier for security? It will depend on the value of the analytic results. That starts with agreeing on, setting, measuring and improving core metrics for your organization, including: false positive and false negative rates, the mean time to detect a threat, and the mean time to resolve a security incident (investigative efficiency). These metrics—and ultimately, the value of your analytic investment—are a direct function of the following components:
1. Data scale. Determining your network baseline requires you to evaluate all your network traffic. Relevant data starts with network flow data, application logs and events, network-based security logs and events (from firewalls and intrusion detection/prevention systems), data from host-based anti-malware tools, information from vulnerability management tools (scanners and patch management), SIEM, log management, and other security detection tools. These tools can generate petabytes of data each day.
Because this data originates from different tools, it is often siloed. Gaining a holistic view of the network requires you to match, reconcile and interpret the various data formats in a manner that does not demand more work from already-taxed security analysts. Correlating this information to provide a complete, temporal picture is a must.
Context is also important for establishing the baseline of network behavior. Context comes from both static information (the department in which an employee works helps define how a specific machine should operate) and dynamic information (typical machine usage patterns that can change over time). Contextual information helps define the “who, what and where” and provides a variety of lenses through which to view a network event. This context provides greater fidelity in normal behavior and dramatically improves anomaly detection.
2. Timing and implementation. Organizations have made considerable investments in SIEM solutions and cyber data lakes that aggregate and index data to provide a centralized view. These solutions typically connect data in a reactive manner, with data going first into storage and correlations occurring later in response to security analysts’ queries. Due to their reactive nature, SIEM solutions struggle with scalability and are not optimized to process or store the data volume necessary to reveal the broader implications of an incident across the organization. All of these factors make it difficult to gain timely and accurate insights from this data.
In order for security analytics to proactively address constantly changing conditions, contextual data needs to be processed in real-time to provide the most accurate baseline. This means correlating information to create a comprehensive picture of network behavior before the data is stored. This results in a more robust data set on top of which analytics can be run and allows security analysts to focus on addressing security risk versus developing the right queries to identify risk.
3. Analytic approach. Security analytics can play a critical role in protecting modern networks. Malicious parties can easily ping a large company’s network millions of times a day. Yet these attacks are rarely noticed because the network may generate billions of events per day. With all of this noise, only the most innovative analytics can enable organizations to find the relatively weak signal.
Despite all the marketing buzz around machine learning, predictive analytics and artificial intelligence, many organizations are only at the beginning of their analytic adoption journey. Typically, companies must progress through a maturity curve before they are able to leverage these advanced approaches. Without a roadmap for analytics use and implementation, organizations will not drive improvement in the key metrics they set.
For many organizations, their ability to become predictive will depend on how accurately they are able to measure their baseline network activity, track detection and investigation outcomes, and feed those outcomes back into an ongoing analytic process. Ultimately, the best solution will be a layered approach that can be deployed against specific targeted activities.
Addressing today’s complex security challenges means adopting sophisticated analytics to detect subtle emerging threats, and as the threat evolves, predictive analytics will be central to ensuring your data assets are properly leveraged.