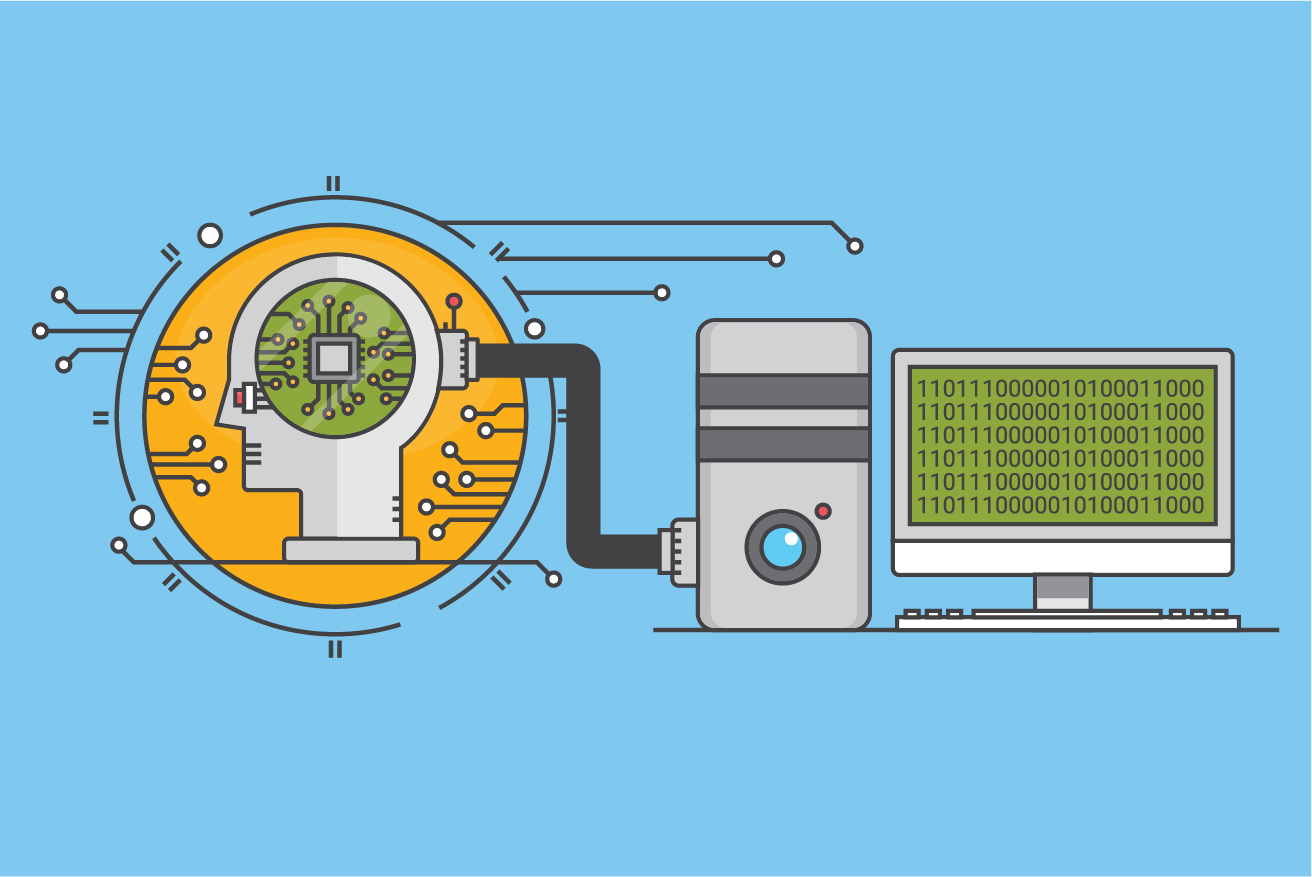
Technology has been a fixture in risk management for quite some time. Credit card companies, for instance, have long leveraged software tools to identify atypical transaction patterns that might indicate fraudulent use of a consumer’s credit card. But as more information becomes available from a wide range of sources, including internal company records, external social media, and email and website data, the uses for technology are expanding as well. In order to make sense of this massive amount of data, more organizations are adopting data science and machine learning tools.
Machine learning harnesses algorithms to sort through large volumes of data collected from structured databases, such as customer or transaction records, and the internet. Data science tools then extract meaning from the data by searching for and flagging correlations and patterns. For example, if risk management is investigating a suspected rogue employee, data science and machine learning tools could be used to perform automated background checks, as well as to analyze the individual’s transactional database activity and social media interactions to find evidence of wrongdoing.
As the capabilities of data science and machine learning have expanded, so have their risk management applications. For example, these tools can help investigate fraudulent insurance claims by looking for clues on claimants’ social media pages and other online activities. Companies have also started using the technology to identify patterns and information that may signify other fraudulent activities. In some instances, data science and machine learning have been used to scan websites to check for intellectual property infringement. The technology is also being used by some operations to identify adverse criminal, regulatory or market developments occurring within companies with which they are partnering or pursuing a merger or acquisition.
Data science and machine learning also allow organizations to more easily collect and access “legally defensible” information, which has become increasingly important, especially in fraud investigations. In order for data to be legally defensible, there must be proof that what was collected is what it purports to be, that it has not been tampered with, and that it was gathered in a manner directed by policy, rather than arbitrarily. Data science and machine learning solutions enable legal defensibility by collecting every request a browser makes to load a page and every response returned directly from the server, hashing and time-stamping them separately, and storing them in a designated container. These solutions can also produce snapshots of pages of data as they appeared at the time of capture, and collect visible links, text and metadata from webpages, among other functions.
Companies are also utilizing data science and machine learning solutions to monitor structured data from their own systems. Potential cash-flow risks resulting from money laundering or embezzlement schemes can then prompt proactive investigation before they become more significant problems for the business. Similarly, in-house counsel does not have to wait until threats present themselves to the organization before responding. For example, technology can help bring to light changes in the behavior of important international supply chain partners (indicated when the technology discovers new terms and conditions or relevant local news coverage) and the appearance of privileged information in the public sphere, which could point to intentional or unintentional leakage of intellectual property.
Implementing Data Science and Machine Learning
While the use of data science and machine learning can have a number of benefits, risk managers will need to work closely with their IT departments to implement such tools effectively. Typically, individuals on the business side have a tendency to shy away from technology-centered projects, believing that the IT department is solely responsible for those issues. But the potential of data science and machine learning tools cannot be fulfilled without intervention from those who run the business. These domain experts are the ones who know which functions the tools should perform in their individual organizations; those in IT know how to make the actual performance happen.
Risk professionals should take steps to inform the implementation process. The first step occurs prior to deployment, before IT configures the technology, when risk managers specify the risks they are attempting to address and which factors they believe are feeding it. They may express concern, for example, about the risk posed by working with a particular business partner, citing concerns about previous relationships with competitors and possible engagement in fraudulent activities.
The second step, executed as the system is being designed, involves supplying the IT department with “training data”—information to be incorporated into the system for the purpose of training the system to uncover patterns. For instance, if money laundering by a business partner is a concern, risk managers might provide information about the circumstances of other cases in which money laundering was discovered. In most instances, risk managers will continue to share training data in order to refine the system over time.
Finally, risk professionals need to offer feedback about the system to their IT department colleagues after its deployment. If the desired information and data correlations are not being uncovered, adjustments can be made to refine the system for better results.
No matter the industry or environment, an organization’s initial foray into harnessing data science and machine learning should not involve a major project that crosses business lines. It is far better to select a particular business process for a trial run and assess the results before undertaking an enterprise-wide deployment. This will make it easier to determine whether adjustments to the system are necessary or if additional information should be added to the platform.
Historically, companies have accessed and used data generated by and stored within their own systems for risk management purposes. Recent years, however, have seen a proliferation of other types of data, including not only unstructured web data from social media pages, but also information exchanged via collaboration tools, emails, websites and the like. As a result, new and improved data analysis tools like data science and machine learning are necessary to sift through it all to enable an organization to identify ongoing fraudulent activity and proactively respond to risks.