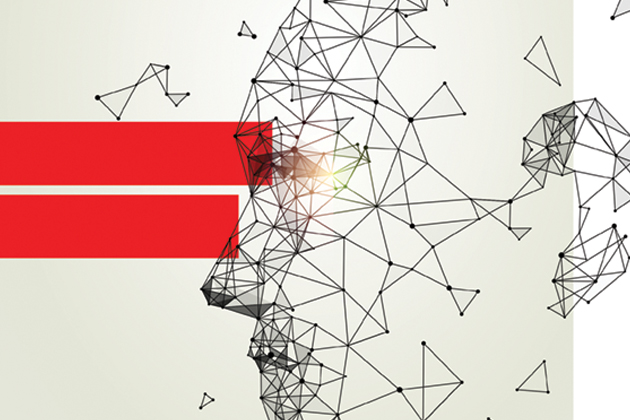
While millions of people happily use facial recognition to log in to their smartphones, 2019 has seen a notable backlash against such biometric technology. Around the world, law enforcement agencies have been criticized for using facial recognition technology to identify potential criminals in public places. In the United States, cities such as San Francisco and Oakland have moved to ban police from deploying the technology over fears that it paves the way for potential privacy violations and mass surveillance.
Even as the public becomes more aware of the risks, many businesses continue to quietly introduce facial recognition technologies. Corporate office spaces, mobile phones, online platforms, airports and shopping centers are all rapidly growing their use of increasingly sophisticated cameras linked to algorithmic software. With the proliferation of devices packed with visual sensors, the ability to capture, analyze and store human faces is expected to increase exponentially.
In its 2019 facial recognition market report, Allied Market Research forecast that the total value of the global sector will reach $9.6 billion by 2022—a compound annual growth rate of 21.3% from 2016. Investment in more sophisticated types of 3D facial recognition devices has accounted for some of that growth as retailers and others look to increase returns on investment by arming existing security systems with facial recognition capabilities. “By assessing customers’ facial expressions and even bodily responses, retailers are able to gain better insights into consumer behavior, even to the point where they can predict how and when a buyer might purchase their products in the future, which will help increase sales,” said Supradip Baul, assistant manager of research at Allied Market Research.
Not So Easy
Despite the growing commercial interest, there are many challenges involved in properly implementing facial recognition technology. Real-life deployments are generally susceptible to failure, especially when used in public places. In 2018, for example, the U.K.’s Cardiff University evaluated South Wales Police’s use of automated facial recognition, analyzing the risks from using cameras to take images of people in crowds, subjecting them to algorithms that created a unique digital “fingerprint” of each face, and then matching them in real time to a database of suspects.
The system was beset with problems that highlight the difficulty of using algorithms to enhance any existing closed-circuit television security system. First, without clear, unambiguous images to compare, the system failed. It was often inaccurate to the extent that it identified some people in the street multiple times as matches, even though they were not in the suspect database. Another issue was that the test used two algorithms that produced drastically different results—a problem that could jeopardize the use of the technology as evidence in court. Finally, the police underestimated the level of training required for personnel to become effective, so costs escalated.
“This is not a take-it-out-of-the-box technology that just plugs and plays,” said Professor Martin Innes, director of the Crime and Security Research Institute and the Universities’ Police Science Institute at Cardiff University. As co-author of the report, he believes that the key finding was just how many factors can radically influence system performance, both in terms of the specific cameras, algorithms and environmental conditions and in terms of the organization and culture of the enterprise adopting such tools.
Controlled Environments
The only way to mitigate these risks is to deploy facial recognition systems in highly controlled environments. Many businesses take this route for identity management and authentication applications, but too often rush in without thoroughly assessing the risks of potential failure. “Businesses see facial recognition systems as easy to use because the demos are so good,” said Nelson Cicchitto, chairman and CEO of identity and access management company Avatier Corporation. “If the demo goes through the enrollment process, it is usually in a quiet environment with good lighting and with people who don’t have bandages on their faces, or a whole host of other potentially problematic factors.”
Cicchitto believes scaling any system beyond about 10 people is difficult. For example, he recently worked with a large U.S. media and communications company that wanted to implement facial recognition for identity and authentication management for its 35,000-person staff. The pilot phase targeted 6,000 people, but the project never got off the ground. “What killed the project for us was the time it took doing the enrollment, getting CIO support, rolling out the importance of the enrollment process to the executives, communicating the benefits that it would make users more secure and, once rolled out, create more convenient working practices,” he said.
The facial recognition implementation strategy has to be completely aligned to a company’s risk appetite and its specific needs, he added. One of the main strategic considerations is the trade-off between convenience and security. More security tends to mean less convenience in the enrollment and authentication process and vice versa. Apple introduced facial recognition technology into its iPhone X in 2017, for example, not primarily as a way to boost security, but to offer greater convenience. If it had tighter recognition controls for authentication, users could quite easily be locked out of their own devices, defeating the goal of greater convenience and thus discouraging its use.
On an operational level, it is critical to get the facial recognition enrollment process right. Having human resources and others involved in marketing the right messages internally can help mitigate some of the concerns. Additionally, ensuring enrollments are conducted in well-lit environments that isolate each person should eliminate the risk of a person logging in to the system with a different identity. Advanced 3D and machine learning processes in some versions of the technology are also more forgiving of people wearing glasses or growing beards over time, and will not lock users out for such changes. They are also less susceptible to access attacks, such as showing a system photographs or videos of an employee to grant access.
Fraud is also tough to prevent in large-scale deployments where a person could pose during enrollment as someone else to gain access to sensitive data and systems. Getting assurance about risks like this is very difficult in a scenario that involves thousands of people. “The reason such biometrics are not used everywhere is because it is designed for a specific culture, a specific type of environment—and the benefits are only available if those elements are in place,” Cicchitto said.
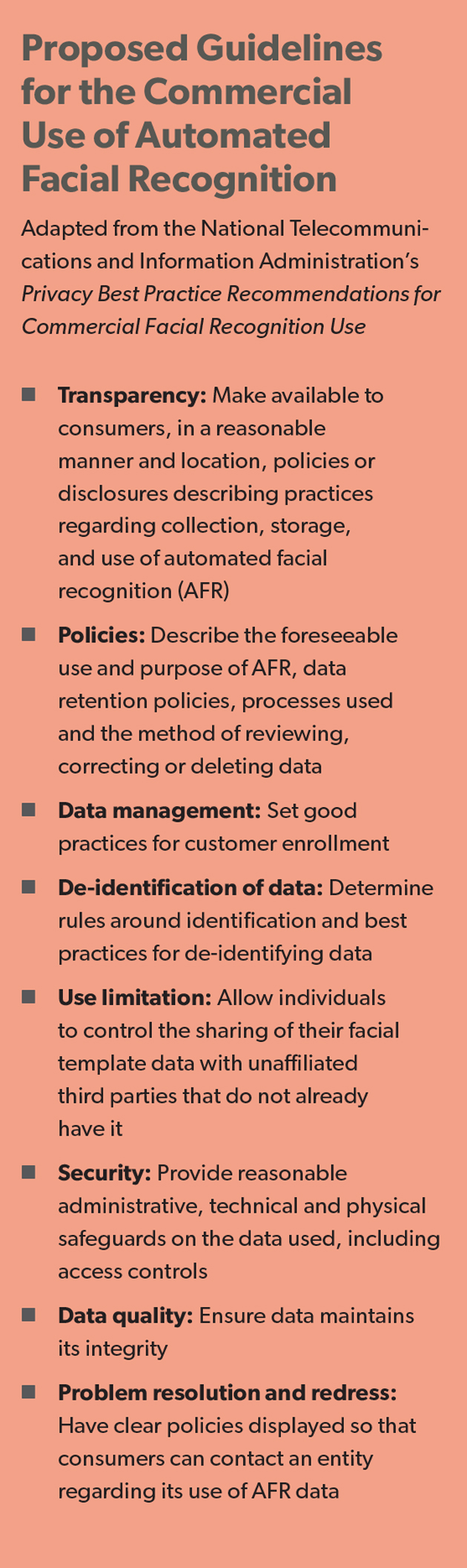
Adopting facial recognition technologies tends to be expensive in terms of both technology and time. According to Grant Thornton cyberrisk principal Rahul Kohli, value-for-money studies need to address how much customer experience and security can be enhanced, and due diligence must be approached more systematically than for other types of technology. In one example, Kohli worked on a public sector project to improve user experience and security with such a system, and although the technology seemed to make sense and the risk assessments looked fine, the customers rejected it. The organization’s due diligence had followed a traditional path by testing the water using small sample groups, but these turned out not to be representative of the entire customer population. Even if a whole sample assessment goes well, small pilot projects are essential to see how the systems work in real situations.
“One reason is to test adoption among actual users, but another is to see how the organization copes with a relatively less-risky piece of, say, intellectual property being exposed on biometrics,” Kohli said. “It allows the organization to do further risk assessments after the fact and start onboarding more applications and platforms using that particular biometric authentication.” Often, organizations get around those risks by only adopting the technology in the most risk-sensitive part of a business, leaving other security provisions untouched. “We don’t advocate a big-bang implementation as they have a tendency to end up with an explosion,” he said.
Privacy Concerns
Facial recognition technologies are gaining traction at the same time that tough new privacy laws are coming into force. The U.S. National Telecommunications and Information Administration’s guidance on the commercial use of facial recognition technologies provides some guidelines (see sidebar on page 22), but provisions of the European Union’s General Data Protection Regulation (GDPR) and the California 2018 Consumer Privacy Act may stymie new commercial projects.
“GDPR is important because the capture of someone’s facial features is personal data if you are able to identify the individual,” said Anita Bapat, a data protection and privacy partner at law firm Kemp Little. As a result, organizations that wish to use facial images usually need explicit consent to do so. Consent has to be unambiguous, freely given and fully informed, and it has to be as easy to give as it is to withdraw. Secretly sweeping up identifiable faces in a shopping mall is unlikely to comply with those laws, or win over customers who discover it has been done without their knowledge and consent. “It is vital that organizations assess the privacy risk of using this type of technology,” she said. “That entails looking at each principle of privacy law that applies to you, what the risk is, and how you can mitigate that risk.”
New Directions
Bapat believes that organizations may be too hung up on faces. “Culturally, we attach a lot of importance to someone’s face in terms of their identity—that is partly why the debate is so heated—but you may be able to use different body parts, clothing, or just one part of the face to draw the same inferences while mitigating the privacy risk,” she said. For instance, if a company wants to advertise to women working in a city’s financial district, they can instead target people wearing certain types of footwear and still reach most of the same demographic. Ultimately, being clear about how and why businesses are using the technology is vital to its acceptance, she said.
The risk of being accused of spying on customers could also blind businesses to some potential benefits of more advanced uses of facial recognition technologies. For example, emotional analytics company Realeyes has been helping advertisers code the attention levels and emotional reactions viewers have to their campaigns. Typically, 200 to 300 participants are paid to take part through their own computer webcams or mobile devices. They must consent to having their reactions analyzed in real time as they watch videos, and that data is anonymized and aggregated on Realeyes’ cloud servers and not shared with third parties. Media executives use the data to see, for example, if there is a dip in attention at a particular point in an advertisement. They can then alter the media or just push their most effective videos while dropping the others.
Over the past 10 years, the company has reportedly built a database of over 450 million frames of faces that have been tagged for six basic emotional responses. Bias is eradicated by using real people to confirm responses tagged by its algorithms. “It is critical to use a lot of people,” said Realeyes CEO Mihkel Jäätma. “Cultural sensitivity is very important—you need Asian people, for instance, annotating Asian emotions because they are going to be more accurate in assessing whether a person is confused or happy.”
Jäätma has no problem with GDPR-type privacy laws and emphasizes their transactional nature. Organizations using facial recognition technologies must have a clear value proposition to offer customers. Realeyes pays people for consent but is now developing other potential reward mechanisms for its fledgling wellness and education initiatives. In Japan, for instance, loneliness and depression have become important social issues. With people spending so much time on mobile devices, apps based on emotional analytics could detect early signs of depression and help users manage those conditions better. The project is in its early stages, but Jäätma is convinced that building a fair and transparent value proposition into the system from the outset will be critical to its success. “It’s just the right thing to do,” he said.