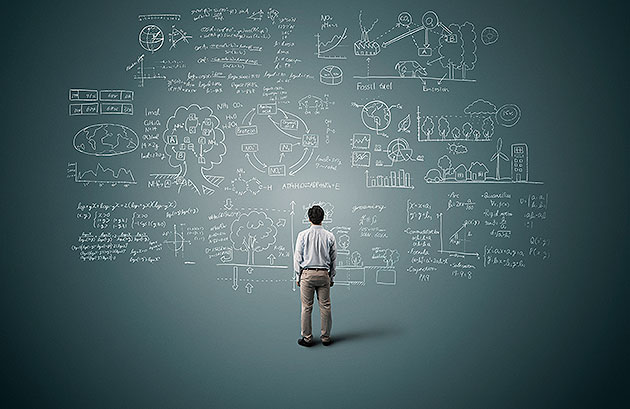
Predictive analytics is a practice that applies statistics, advanced mathematics and a mix of technologies to analyze large quantities of data and past events to identify patterns and build forecasts about future events. It has been supercharged by techniques that include crowd-sourcing, machine learning capabilities-the ability of machines to learn without being programmed-and improved big data aggregation technologies. Some predictive analytics firms also offer platforms that tout easy-to-use interfaces designed for a broader base of users than just data scientists.
"Traditionally, predictive analytics was thought of as something utilized by a secret group of specialists locked away in a room," said Paul Ross, vice president of product marketing at Alteryx, a four-year old predictive analytics startup based in Irvine, Calif., and backed by SAP Ventures and Thomson Reuters. It helps a range of companies-including McDonald's and Time Warner Cable-analyze customer preferences, choices and customer attrition risks.
Platforms such as Alteryx's, however, are also making it easier for everyday people to access the predictive analytics process. "[Predictive analytics] will now be in the hands of more organizations, with more and more people on the frontlines of business adopting its use," Ross said.
Indeed, while only 13% of companies had a predictive analytics system in place in 2012, research firm Gartner projects that 70% of the most profitable companies will employ either predictive analytics or more real-time collaboration techniques to ensure competitive advantage by 2016.
Risk management teams are particularly seeking out these capabilities. A global survey conducted by the IBM Institute for Business Value found that 47% of organizations across industries now use predictive analytics to support business insight for risk purposes. "I was personally impressed with how many are using predictive analytics for risk management," said IBM strategy and analytics expert Karen Butner.
"Risk is one of those areas that is a key beneficiary of advances in predictive analytics due to the ability to identify and predict vulnerabilities, instances of fraud, security breaches and the quality of control systems and governance," said Rita Sallam, a research vice president and analyst at Gartner. "It is also one area where companies have high intentions, over the next several years, to invest in the technology and increase their usage."
The Winning Solution
One factor that may be limiting broader use, however, is the shortage of data scientists-people with specialized training who understand the predictive techniques and model types-and can help others make savvy use of some of the more sophisticated tools.
San Francisco-based start-up Kaggle aims to address this issue through a type of data scientist outsourcing. The four-year-old, venture-backed firm hosts online competitions for its community of more than 150,000 data scientists in the academic, corporate and government arenas from over 100 countries.
Scientists who join the community are invited to compete in a range of competitions that involve building the best predictive model for large quantities of data. In doing so, they aim to answer complex questions such as: What is the best way to price my product for a targeted audience? What is the best way to identify financial fraud at my sprawling, global organization? What is the best way to predict insurance claims based on car characteristics? And even, what is the best way to measure the shapes of galaxies?
The competitions usually last two to three months. In addition to the opportunity for recognition and the thrill of discovery, participants are motivated by cash prizes-anywhere from $3,000 to $3 million for the best algorithm. According to Karthik Sethuraman, Kaggle's head of analytic solutions, "For many of the data scientists who participate, it's a way to benchmark themselves among their peers around the world."
They also get to tackle a difficult problem that might not ordinarily come their way. For example, it was a data scientist specializing in glacier analysis who solved the galaxy question for NASA. Kaggle has also started hosting recruiting competitions where the winning prize is the opportunity to interview for a job at the organization sponsoring the contest.
The Kaggle competition service tends to attract fairly sophisticated predictive analytics users. Participants include firms that may already have data scientists on board and, in many instances, some that have not been able to improve their own internal risk or predictive models any further, but want to try to squeeze more out of an established algorithm. "For many companies, even a small percentage improvement in a predictive risk model can translate into tens of millions of dollars of profitability," Sethuraman said.
Allstate, Facebook, Ford, GE, Microsoft and Pfizer are among those that have hosted competitions, which can be either public or private. There are usually three top winners to any Kaggle challenge, and private competition clients get the opportunity to own the three new algorithms outright. In the case of a public competition, they can obtain a non-exclusive license.
Kaggle has recently branched out beyond competitions to work more closely with the oil and gas industry. A team of data scientists on staff are developing predictive algorithms to help the energy industry better predict oil and gas drilling outcomes-particularly in hydrofracking-based on the geology of a given property, the equipment, amount and type of fluid and drilling strategy to be used. "To date, the industry does not employ much machine learning or predictive technology, but we think we can help them do so to find the optimal combination of parameters to extract the most amount of oil or gas," Sethuraman said.
Democratizing Analytics
Another new venture-backed entrant to the predictive analytics arena is Seattle-based Context Relevant. Founded by data scientist Stephen Purpura, the two-year-old, 30-person firm is focused on using predictive analytics, machine learning and big data techniques to advance risk management efforts.
"We're faster than the engine that runs all of Facebook," Purpura said. His firm's predictive analytics platform can process 30 petabytes of data per second, allowing for nearly instant model-building results and predictive services like personalized pricing. Customers can get answers to questions in real-time, such as: "How should we price this order in order to win the business and maximize sales?" "What is the bank's comprehensive value at risk (VAR) estimate?"
The firm claims to deliver the world's fastest segmentation and valuation application, which can detect changes in the way customers or assets cluster or diverge. It also says that its technology can facilitate the construction of far deeper predictive models at a very fast rate, thus helping firms achieve a more accurate picture of financial risk.
"Before any trader places a trade, our technology can determine what the effect will be on the institution's assets in real-time," Purpura said. "We have all heard of instances where traders almost brought down the organization. Firms will now be able to know about the possibility of such events before they happen."
Context Relevant's predictive analytics platform can also use encrypted data. "With every other predictive analytics system in the world, you have to push data into the system that is unencrypted, making it vulnerable to hacker attack," Purpura said. "No organization wants to become the next poster child for a major data loss."
According to Julien Sauvage, head of product marketing for advanced analytics at SAP AG, new entrants are democratizing the entire predictive analytics field. Last year, SAP acquired predictive analytics firm KXEN, which will be called SAP Analytics. "What KXEN brings to the table is ease of use and a plug and play format," Sauvage said. He added that the platform's end-user is typically a business person who does not need to know anything about predictive analytics, only the nature of the business problem he is trying to solve. "We are definitely bridging the knowledge gap and making predictive analytics accessible to everyone," he said.
Sauvage believes that financial firms' fraud detection efforts can be improved by combining the SAP/KXEN platform with SAP's in-memory solutions that speed up pattern-matching efforts and its existing visualization tools. "Companies have not focused as much on the growing instances of online fraud, and the dollar-loss amounts are amazing," he said. "These companies need to switch from simply monitoring and predicting fraud on an individual customer basis to also making predictions about every single transaction."
Necessary Feedback
New York-based Opera Solutions, founded in 2004, also provides a package of applications that combine predictive analytics capabilities with aggregated, industry-specific data serving the finance, risk, insurance, health care and supply chain operations sectors.
Its Signal Hub platform combines machine-learning techniques with predictive analytics. As a result, Chief Operating Officer Laks Srinivasan, said that it functions as a learning system. "There is a feedback loop that is constantly looking at predicting based on what is actually happening." With such technology now available, he believes that companies can no longer just build a predictive analytics model and only recalibrate it every six months. Without constant feedback, any predictive analytics system can quickly become stale and will not be very useful.
Srinivasan said that one area where Opera's technology has been especially helpful is financial services and, specifically, in the bond market and with mortgage-backed securities. "Opaqueness is a big challenge in that industry, and so we are able to open up the constituent parts of a bond and look, in an anonymous fashion, at the type of consumers who are part of a particular product, and the credit profile of each one of the borrowers in the pool who are driving the cash flow of the bond-something that was not done before because there was way too much data," he said.
Having access to all this data for predictive analytics purposes allows his customers-many of whom are risk managers and portfolio managers-to be much more predictive about investment outcomes and understand the intrinsic value of the bond, he added.
But as this technology is systematically applied to consumer behaviors and demographics and utilizes ever more data in a range of industries, what will be the outcome? "Whether it is a risk manager, portfolio manager, CEO or marketer, it's all about man and machine working together," Srinivasan said. He believes that in five years corporate management teams will use technology to not only be predictive, but also prescriptive. Ultimately, predictive analytics will be able to tell us more than just where we want to go, it will also tell us the best way to get there.